Discover the groundbreaking AI tool, GraphCast, developed by Google DeepMind, which outperforms traditional weather forecasting models in predicting storm landfalls. Uncover how this innovative technology, producing predictions in less than a minute, could revolutionize our ability to prepare for extreme weather events.
Introduction: In a leap towards unprecedented accuracy in predicting storm landfalls, artificial intelligence emerges as a potential lifesaver. Google DeepMind's revolutionary AI tool, GraphCast, has demonstrated its prowess by forecasting Hurricane Lee's landfall in Canada three days in advance, outshining conventional forecasting methods. As we delve into the realm of AI-driven weather predictions, it becomes clear that the future of storm forecasting is undergoing a transformation.
The Game-Changer: GraphCast's Early Predictions
Faster and More Accurate
Artificial intelligence, through the lens of Google DeepMind's GraphCast, has proven its ability to predict the trajectory of storms with remarkable precision. September's Hurricane Lee serves as a testament, with GraphCast surpassing existing forecasting systems by providing a three-day lead, a crucial advantage in the face of impending natural disasters.

A Shift in the Forecasting Paradigm
Traditional weather forecasting, while significantly improved over the years, faces a paradigm shift with the introduction of AI. The speed and analytical capabilities of GraphCast propel storm predictions into a new era, offering faster insights and enhancing our preparedness for extreme weather events.
GraphCast vs. Traditional Models: A Decisive Lead
Unparalleled Accuracy
Published in the journal Science, DeepMind's paper reveals that GraphCast outperforms the European medium-range weather forecast model, renowned as one of the world's best, in over 90% of analyzed factors. This AI tool, operating with unprecedented speed, produces predictions within minutes, a stark contrast to the substantial computing power required by traditional methods.
Revolutionizing Data Processing
GraphCast's efficiency lies in its departure from traditional data processing methods. While conventional models involve analyzing real-time atmospheric conditions, GraphCast adopts a unique approach. By analyzing past events, it achieves superior accuracy, making sense of vast datasets using a fraction of the computing power required by existing forecasting models.
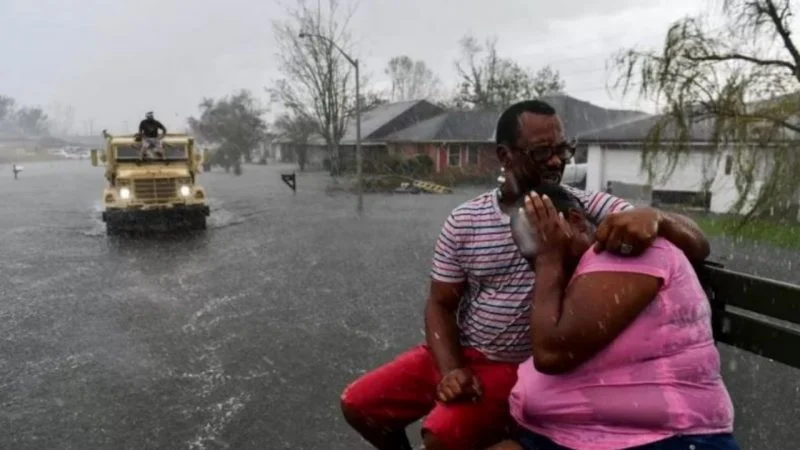
The Complexity of Traditional Forecasting
Millions of Measurements
Traditional weather forecasts involve processing millions of readings from diverse sources worldwide, ranging from weather stations and satellites to balloons and buoys. Matthew Chantry of the European Center for Medium-Range Weather Forecasts highlights the intricate process of selecting the most crucial data among the vast ocean of information, with approximately 10 million measurements influencing a single prediction.
Supercomputing Power
The traditional method relies on supercomputers processing an immense volume of data, performing quadrillions of calculations per second. Complex equations simulate atmospheric changes, contributing to the evolution of weather forecasts over time. While undeniably successful, this method now faces a formidable challenger in the form of AI-driven predictions.
As AI continues to reshape the landscape of storm predictions, GraphCast stands at the forefront of innovation, challenging the conventions of traditional weather forecasting. The implications extend beyond routine decisions to what to wear in the morning, offering communities invaluable time to prepare for and mitigate the impact of storms, floods, and heatwaves. The era of AI-powered storm forecasting is upon us, promising a future where our ability to anticipate and respond to extreme weather events reaches unprecedented heights.
Comments